The Role of Annotation Machine Learning: Unlocking Business Potential
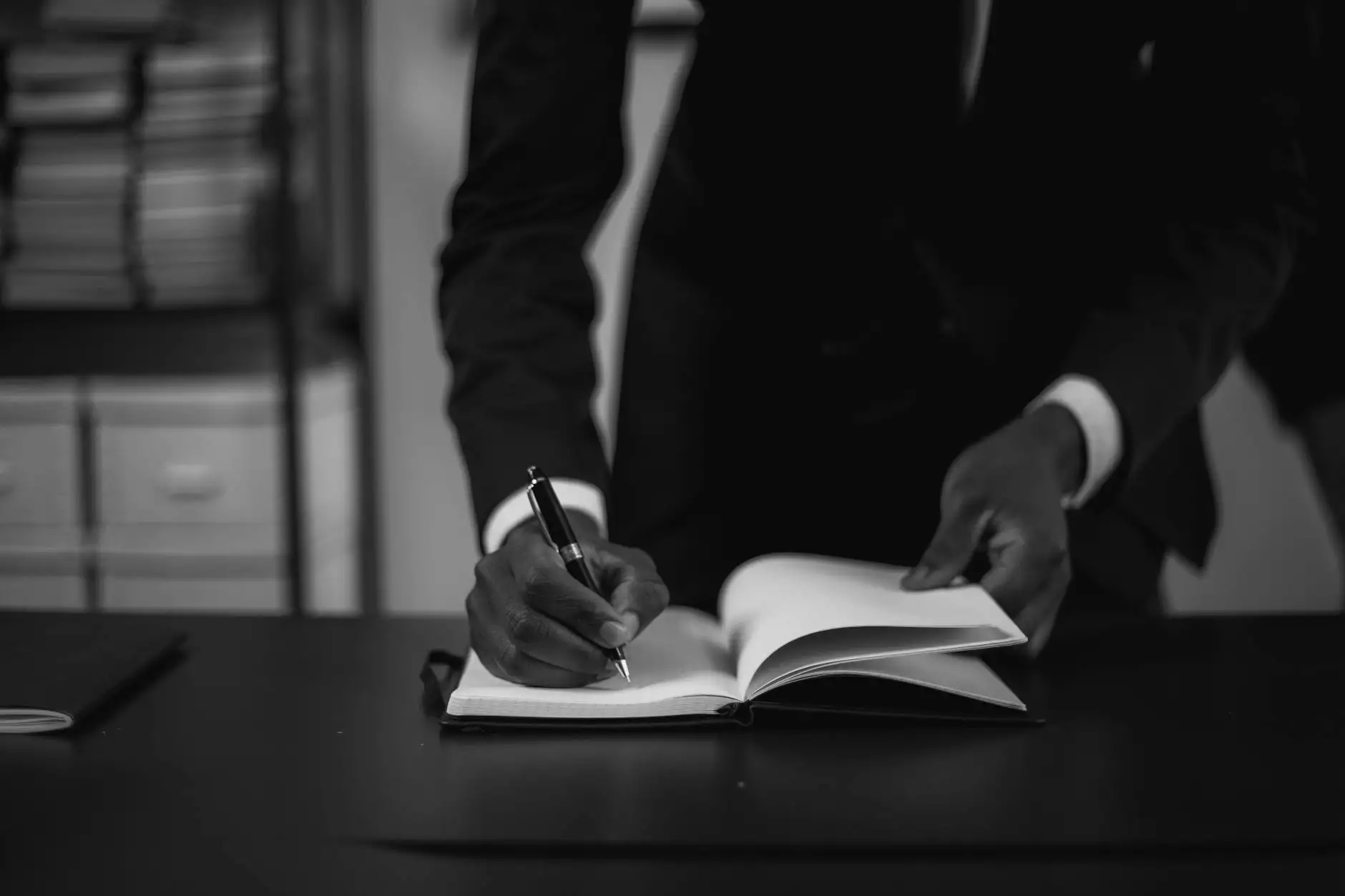
In a world increasingly driven by data, the application of machine learning has become paramount in various sectors, including Home Services and Keys & Locksmiths. A critical aspect of this technological evolution is the process of annotation. This article dives deeply into the concept of annotation machine learning, elucidating its importance, methodology, and impact on businesses, particularly in the realms of Home Services and locksmithing.
What is Annotation in Machine Learning?
Annotation in machine learning refers to the process of labeling data so that machines can learn from it. This is particularly vital in supervised learning, where the algorithm learns to make decisions based on labeled input data. In essence, when data is annotated, it allows the machine learning models to understand patterns and make predictions or classifications based on new, unseen data.
The Importance of Data Annotation
The efficacy of a machine learning model relies heavily on the quality and volume of the data it is trained on. High-quality annotation ensures that the model can interpret and analyze data effectively. Here are some reasons why data annotation is crucial:
- Improves Accuracy: Properly annotated data leads to more accurate model predictions.
- Enhances Understanding: It allows machines to understand context and nuances in the data.
- Facilitates Learning: Models learn better from structured data that has been labeled correctly.
- Increases Efficiency: Annotated data helps in faster training and deployment of models.
Methods of Data Annotation
There are several methods to annotate data for machine learning applications. Understanding these methods can aid businesses in selecting the right approach for their needs:
1. Manual Annotation
This traditional method involves human annotators who label the data. While it can be time-consuming, it often results in high accuracy due to human judgment. For businesses like locksmiths requiring precise definitions of services and needs, this could be invaluable.
2. Automated Annotation
In this method, algorithms are used to tag data automatically. While this is faster and can handle vast amounts of data, it may lack the precision of manual annotation. However, it's evolving rapidly with the advancement of deep learning techniques.
3. Crowdsourced Annotation
This involves gathering a large number of contributors to label data. Platforms like Amazon Mechanical Turk enable businesses to access scalable resources quickly. It is cost-effective but requires rigorous quality control.
Applications of Annotation Machine Learning in Home Services and Locksmithing
As businesses in the Home Services, Keys, and Locksmiths categories adopt machine learning, the role of annotation becomes increasingly significant. Here are some applications:
1. Customer Service Automation
Many locksmith services are now using chatbots powered by machine learning. Annotation helps these bots understand customer inquiries, enabling them to provide relevant responses quickly.
2. Predictive Analytics
Through annotated data, locksmiths can predict customer needs based on previous interactions or location. For example, analyzing call logs and service requests can help anticipate high-demand scenarios, allowing businesses to allocate resources efficiently.
3. Image Recognition for Keys and Locks
Machine learning models can be trained on images of keys and locks. Annotation assists in identifying different types of keys, making it easier for locksmiths to offer precise services. For instance, specific types of locks may require unique keys, and being able to identify this quickly enhances service speed and customer satisfaction.
4. Marketing Targeting
By annotating data gathered from customer interactions, locksmiths can identify key demographics and tailor marketing strategies effectively. This can lead to increased conversion rates as promotional campaigns become more targeted and relevant.
Benefits of Using Annotation Machine Learning in Business
1. Enhanced Decision Making
With the insights gleaned from annotated data, businesses can make informed decisions, reducing risks and improving operational efficiency.
2. Cost Reduction
Automating processes using machine learning can significantly reduce operational costs. Businesses in the locksmith industry, for example, can save on labor while maintaining high service quality.
3. Customer Satisfaction
By personalizing services based on data annotation, businesses enhance customer experiences. A locksmith service that understands client preferences and previous interactions can offer more tailored solutions, resulting in higher customer satisfaction.
4. Competitive Advantage
Annotation machine learning can give businesses a leg up in their market. By leveraging data insights, companies can stay ahead of trends, anticipate consumer behavior, and adapt quickly to changing demands.
Challenges of Annotation in Machine Learning
While the benefits of annotation machine learning are substantial, there are also challenges that businesses may encounter:
1. Quality Control
Ensuring that annotations are accurate is critical. Poorly labeled data can lead to faulty model predictions, which can harm business operations and reputation.
2. Resource Intensive
Annotating data, particularly manually, can be time-consuming and resource-intensive. Businesses must weigh the costs against the potential benefits.
3. Evolving Data Requirements
As markets and technologies evolve, the data needs for training models also change. Continuous annotation may be required to maintain accuracy over time.
Best Practices for Successful Annotation
- Define Clear Guidelines: Establish clear and comprehensive annotation guidelines to ensure consistency.
- Utilize Multiple Annotators: Having multiple people annotate the same data helps to identify discrepancies and improve accuracy.
- Regularly Review Annotations: Implement a review process to maintain the quality of your annotations.
- Leverage Technology: Use advanced tools and software to facilitate the annotation process, whether manual or automated.
Conclusion
The integration of annotation machine learning into business models presents immense opportunities, especially in the Home Services and locksmithing sectors. By properly annotating data, businesses can enhance their operations, improve customer engagements, and ultimately gain a competitive edge in an increasingly data-driven marketplace. As machine learning technology advances, the importance of quality annotation will only grow, making it an indispensable aspect of modern business strategy.
As the landscape of Home Services continues to evolve, businesses that invest in robust data annotation efforts will be well-positioned to thrive in the future. Embracing these technologies is not just a trend; it’s a necessity for sustained growth and success in the competitive marketplace.