The Power of Bounding Boxes in Data Annotation
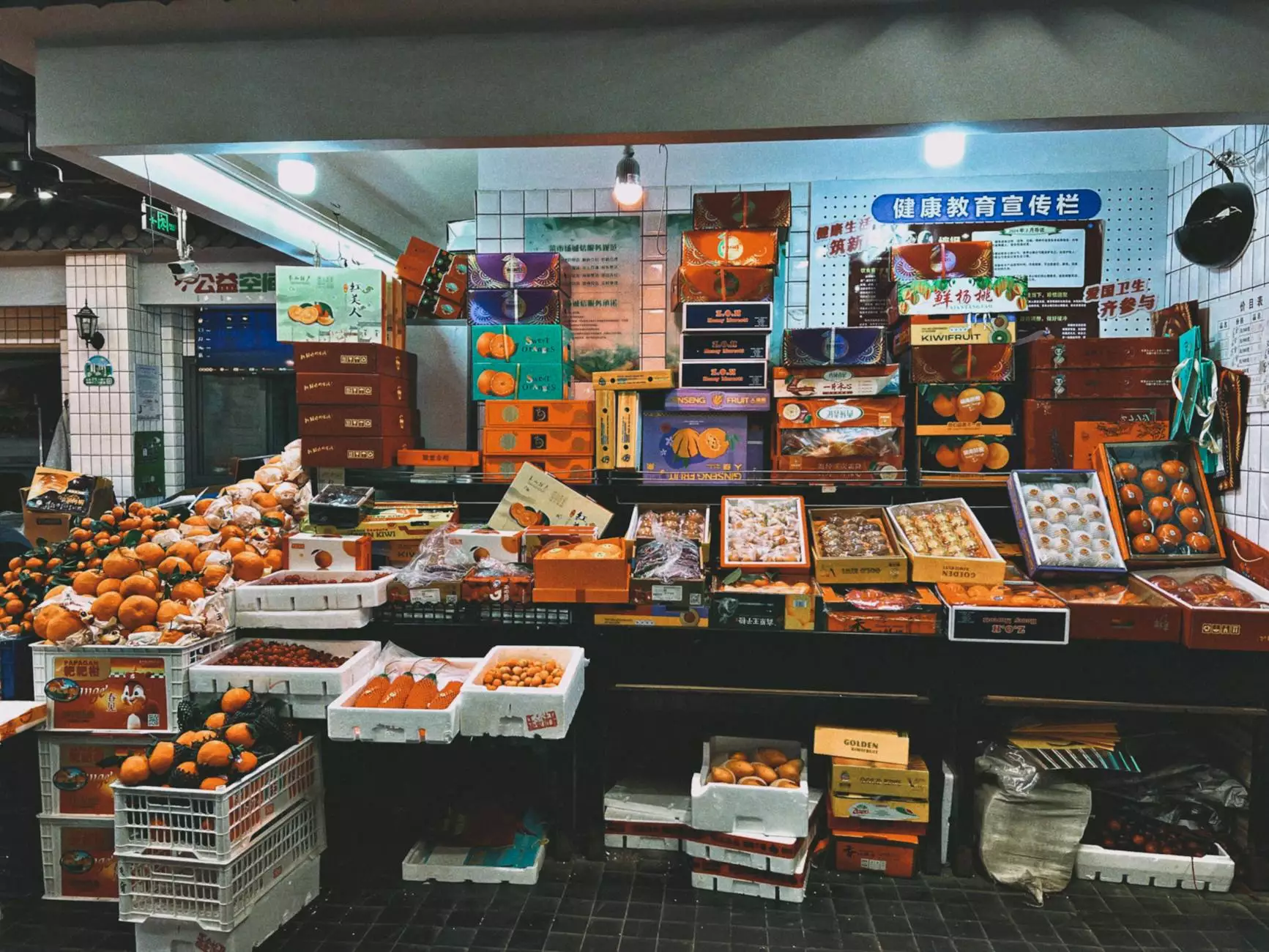
In the era of artificial intelligence and machine learning, data is the new currency. The ability to harness the power of data through effective annotation tools is vital for the success of machine learning models. One pivotal concept in this domain is the bounding box, a simple yet powerful tool that has revolutionized how we perceive object detection in various fields, from autonomous driving to healthcare diagnostics.
What is a Bounding Box?
A bounding box is defined as a rectangular box that encloses an object within an image or a video. Its primary purpose in data annotation is to identify and localize the target object, which can be images of cars, animals, humans, or any other significant entity relevant to the dataset at hand. The bounding box is generally represented by its coordinates, which denote the edge's position and size, typically in pixels.
The Importance of Bounding Boxes in Data Annotation
Understanding the significance of bounding boxes is crucial for organizations looking to enhance their data annotation processes. Here are several reasons why they are integral:
- Object Localization: The primary function of a bounding box is to help a machine learning model accurately locate and recognize objects within an image, allowing for better predictions.
- Facilitating Training and Validation: Bounding boxes are essential during the training phase of machine learning models, as they provide clear data for validating the model’s performance on object recognition tasks.
- Scalability: As businesses scale their data requirements, using bounding boxes provides a systematic way to uniformly annotate large datasets, ensuring consistency across all entries.
- Enhanced Object Detection Capabilities: With well-defined bounding boxes, models can achieve higher accuracy in detecting and classifying objects, leading to improved outcomes in real-world applications.
How to Create Effective Bounding Boxes
Creating effective bounding boxes is not just about drawing rectangles around objects; it involves a nuanced understanding of precision and context. Here are some guiding principles:
1. Understand the Object’s Context
Always consider the object’s surroundings. The bounding box should encompass enough of the object while minimizing background noise.
2. Consistency is Key
Ensure uniformity in the size of the bounding box across similar objects to facilitate training. This consistency leads to better model learning over time.
3. Highlight Key Features
When annotating, always focus on the most critical features of the object. For instance, while annotating a car, include not just the body but crucial elements like wheels and windows if they distinctly categorize the object.
4. Utilize Annotation Tools
Leverage advanced annotation software tools provided by platforms like KeyLabs.ai, which offer intuitive interfaces for creating accurate bounding boxes efficiently.
Technological Advancements in Bounding Box Creation
The field of data annotation is continually evolving, and so are the technologies surrounding bounding box creation. Key advancements include:
- Automated Annotation Tools: Many modern tools are now capable of semi-automating the bounding box creation process using AI algorithms that learn from previous annotations.
- Real-time Annotation: With the integration of video annotation capabilities, tools can now draw bounding boxes in real-time, allowing for quick data collection in moving environments.
- Improved User Interfaces: Modern annotation platforms prioritize user experience, enabling quicker annotation without compromising accuracy.
Challenges in Bounding Box Annotation
While bounding boxes provide robust solutions, they are not without challenges. Here are some common issues faced by data annotators:
1. Occlusion
When objects overlap, it often results in bounding boxes that do not accurately represent the true position or size of objects. This requires careful judgment from annotators to deal with occluded objects effectively.
2. Scale Variability
Objects of differing sizes can pose challenges. Large items may dominate the image, leading to disproportionate box sizes, while small items may become difficult to annotate accurately without minutely zooming in on them.
3. Manual Errors
Human errors during manual annotation can lead to imprecise bounding boxes. Training annotators and employing machine learning models to assist can help mitigate this risk.
Best Practices for Optimizing Bounding Box Annotation
To ensure the effectiveness and accuracy of data annotation involving bounding boxes, practitioners should follow these best practices:
- Round Training for Annotators: Provide extensive training for your annotators to ensure they understand the best practices for creating bounding boxes.
- Regular Quality Checks: Implement routine audits of annotated data to ensure that the bounding boxes meet required standards.
- Utilize Feedback Loops: Create mechanisms for annotators to receive feedback on their work so that they can continuously improve their techniques.
- Collaboration with Data Scientists: Work closely with data scientists to draw on insights that can improve the annotation process, particularly for complex datasets.
Applications of Bounding Boxes Across Industries
Bounding boxes find applications across various sectors, each reaping the benefits of improved data annotation:
1. Autonomous Vehicles
In autonomous driving, bounding boxes are crucial for detecting pedestrians, other vehicles, and road signs, enabling safer navigation through complex environments.
2. Healthcare
In medical imaging, bounding boxes assist in identifying tumors or anomalies within diagnostic images, supporting radiologists in making informed decisions.
3. Retail and E-commerce
Bounding boxes can be used in the retail sector for facial recognition technologies or to track customer behavior within stores through video analysis.
Future Trends in Bounding Box Annotation
The future of bounding box annotation looks promising as advancements in technology continue to unfold. Here are some forecasted trends:
- Integration with Augmented Reality (AR): As AR technologies improve, bounding box annotations might evolve to be more interactive and intuitive, assisting users in real-time object identification.
- AI-assisted Annotation: The role of AI is expected to expand beyond mere automation to assist human annotators actively, learning and predicting bounding box placements based on context.
- Data Privacy Enhancements: With increased regulatory scrutiny over data privacy, techniques for anonymizing data annotated with bounding boxes will become more refined.
Conclusion
Bounding boxes are much more than mere rectangles; they are a foundational element in the realm of data annotation that lays the groundwork for superior machine learning initiatives. As organizations strive to harness the full potential of their data, the importance of precise and efficient bounding box annotation cannot be overstated. With innovative solutions and methodologies offered by platforms like KeyLabs.ai, businesses can optimize their annotation processes to ensure they are at the cutting edge of technology.
By focusing on best practices, leveraging technology, and understanding the challenges and applications of bounding boxes, organizations can significantly enhance their data annotation protocols and, ultimately, their machine learning outcomes.